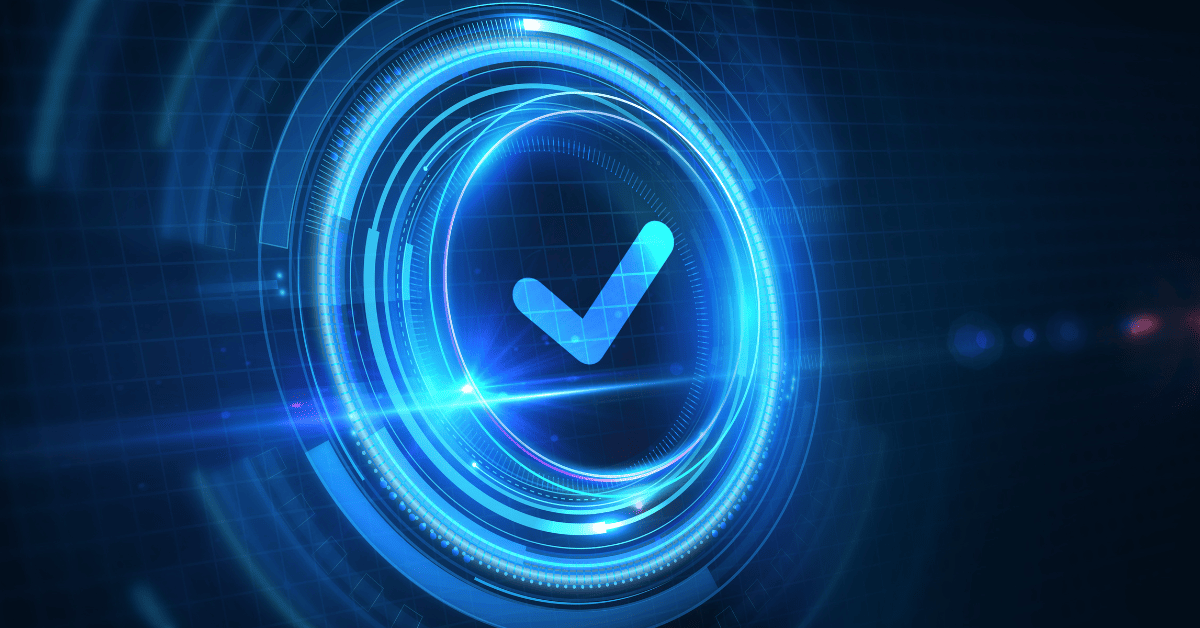
April 1, 2022
There is an adage in machine learning: garbage in, garbage out. Customer experience management platforms like Nexcom’s RevealCX Boost ¹ offer innovative ways of efficiently collecting data. Artificial intelligence (AI) has vast potential and is lucrative, but rushing to implementation can be a recipe for disaster unless we eliminate biases first. How can we design a function that minimizes the possibility of AI delivering the wrong results?
Quality Assurance Sampling Practices
Historically, quality assurance (QA) has been a money pit for contact centers and customer experience organizations. Typical QA programs provide a limited view into a small sample of customer transactions and do not have a measurable impact on the customer experience or key business results.
QA primarily evaluates agent performance and provides information for assessing and training agents. By analyzing the controls in place, we can find a solution to ensure consistent performance evaluation.
Certain QA practices have been questioned. There are concerns about the ability to evaluate thousands of transactions daily or hourly. It takes a small army of quality evaluators to gain real customer experience insight and achieve significant performance results.
QA sampling practices often have good intentions but expose the data to bias by focusing only on certain types of transactions, such as duration. Once we introduce sample bias, we affect the usefulness of the data in making broader decisions.
Without the appropriate controls, there is a risk of compromising data integrity. Whether we use the information to coach and manage agent performance or for broader decision-making — unreliable data adversely impacts our confidence in taking subsequent action.
Connecting Quality Assurance to Business Directives
It is often unclear what direct effects QA has on higher revenues, better business performance, and other financial metrics. The lack of correlation causes businesses to shift QA to the bottom of the payroll or abandon it altogether.
Those that keep QA are not typically leveraging the core function to its full potential. Of course, we support the role of QA in a well-operating organization, but it must drive business value.
High-performance organizations have figured out two key things:
- Incorporating Best Practices into QA design
- Using the right tools to address gaps
When designing a QA function, we first look at ways to establish a connection with the things that matter to the business. In other words, how do we draw the line from QA to critical business metrics and the people we are trying to reach?
We start by gathering and analyzing different types of customer data, such as sentiment, expectation and preferences. With this type of data, customers teach us what matters most, what motivates them to buy our products and grow their loyalty.
Customer information serves as the foundation for designing a QA function that directly influences vital Key Performance Indicators.
Critical Errors and Process Performance
When we fail to carry out processes that support drivers of satisfaction and loyalty, it is a Critical Error ². Successful businesses invest significant time rooting out the cause of a Critical Error and implementing far-reaching, systematic solutions for sustainable performance improvement.
Effective organizations gather data to evaluate individual performance and the entire service journey. They hone support model design and processes that affect customer experience using the proper tools to pinpoint issues and identify opportunities.
Optimizing every aspect of the service journey involves finding and validating the behaviors (human and digital) that drive higher customer satisfaction and loyalty.
Common drivers of satisfaction:
— Resolution accuracy
— Speed of service
— Customer effort
— Empathy
The first aspect of closing service gaps is knowing which behaviors or service elements to evaluate. Next is knowing where to reduce individual agent errors and fix the process. Incidentally, having a massive team of quality evaluators is not economically viable for contact centers and forces them to take questionable sampling approaches.
Accurate and Representative Data
AI opens the possibility of analyzing swaths of transactions from the whole population without biased samples. It seems like a promising customer experience management solution. It increases efficiency while reducing costs and enables companies to quickly identify whether issues are agent—or process-related.
However, it is necessary to vet the information we use to train the machine. AI offers new and improved ways of collecting vital CX-related data. The stakes are high; any biases we pass on to AI can have severe consequences for operations.
Incorporating best practices ³ within every aspect of a QA program, beginning with form design, helps minimize the possibility of AI delivering the wrong results.
Identify and Fix Root Causes VS Defining Problems
Customer experience management (CXM) tools like RevealCX Boost have the potential to free your staff’s time, enabling them to fix issues rather than define problems. RevealCX can show how agents perform and what impacts the customer experience. At the same time, AI can only monitor what is said or done in an interaction. You will still need to manage, recruit, train, coach, develop, and motivate your staff.
Potential for Generating Large Data Sets
Gathering data that used to take a month now only takes a day. With proper calibration, tools such as RevealCX give you data you can trust. There is no unintended bias that affects the usefulness of sample data, which allows you to make final decisions with greater confidence.
Leveraging Customer Interactions with Business Intelligence
Imagine evaluating an agent based on the entirety of their customer communication for a set period versus a few interactions. Using numerous transactions and precise accuracy rates, AI is a practical way of assessing agents and facilitating training.
By looking at a significant number of samples, you can improve the quality of coaching you provide. Instead of saying to an agent, “We noticed you made a mistake on one of the four calls we analyzed,” it would become, “You have made X mistake Y number of times during the last month; let’s work together to fix this.”
Did you know that:
— 75% of issues are process-related
— 25% are agent-related
Getting AI up to a 90% Accuracy for Agent Performance Evaluation
The number of evaluations an organization can create is the limiting factor in accumulating enough data to train a QA artificial intelligence system. Time scales will vary depending on the size of the operation.
Nexcom’s Chief Technology Officer, Iain Ironside, said, “From our experience so far, we believe that once we have around 1,500 evaluations with a good representation of transactions with both errors and acceptable handling, we can start to create models with an acceptable level of accuracy.”
With a substantial QA team, large operations taking thousands of transactions will rapidly build the database. On the other hand, it will take more time for a small group with only a couple of quality evaluators to establish a database for machine training.
Voice transactions require an extra step for implementation. Transaction analysis will inevitably be a more extensive undertaking because this stage involves capturing sentence structure. There is not much time difference after turning the transaction into text for the machine.
Organizations will quickly get more precise answers and reduce costs by investing time upfront.
Room for Humans
Customer satisfaction surveys and focus groups remain the primary means of data analysis for validating the main drivers of customer satisfaction and loyalty.
Even when AI can interpret voice interaction, experts will invariably have to classify what is positive and negative for machine learning. Iain Ironside said there’s still a need for a gauge, an expert who will ensure the machine is evaluating correctly.
Knowledge Changes
With more customer voice analysis, operations will inevitably change their definitions of voice attributes. In the same way we retrain a human monitor, a machine-learning model needs to be retrained. The retraining cycle will be vital and lead to another area of study.
Our Stance on Artificial Intelligence
AI-driven customer experience management software can be a practical way to influence Key Performance Indicators across customer support. CXM platforms can help organizations meet expectations and build loyalty by using data to understand what matters to customers.
In short, AI does not eliminate the need for humans or Best Practices. If you have an incorrect form with a faulty design, AI will make inaccurate evaluations, and you will still go in the wrong direction—just at a faster rate.
For more, watch The Impact of Artificial Intelligence on Contact Center Quality webinar.
- Critical Error: anything from the customer’s perspective that causes the transaction to be defective, such as not solving the query (whether this necessitates a repeat transaction), mistreating the customer, and failing to communicate clearly
- Best Practice: COPC Inc.’s first-hand experience from audits and reviews conducted around the world and across industries and/or business sectors. This is the best approach, process, or method witnessed by COPC Inc. to address either a particular requirement of the COPC CX Standard or a process performed in a customer contact operation.