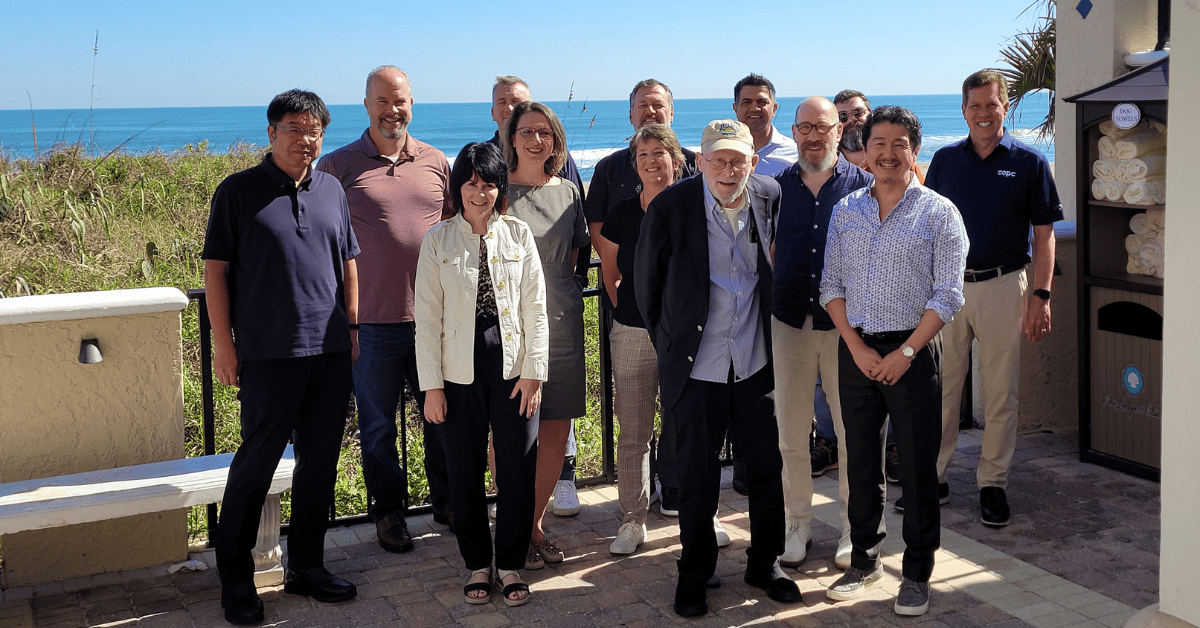
February 15, 2024
BACKGROUND
The COPC Standards Committee is a group of industry leaders composed of the largest buyers and providers of customer experience (CX) operational services, including contact center support. Its role is to maintain the best practices and requirements in the COPC CX Standard.
The COPC Standards Committee meets semi-annually to update the Standard. In a recent meeting, the committee reviewed the impact of artificial intelligence (AI) on CX operations and end-user customer satisfaction.
This paper is intended for current and prospective users of the COPC CX Standard. It presents the COPC Standards Committee’s observations on the application of AI in CX and the approach to determine potential changes to the Standard. Additionally, it examines the terminology used in the Standard and identifies emerging metrics that are candidates for inclusion. Lastly, it announces the establishment of subcommittees dedicated to continuously evaluating AI utilization.
OBSERVATIONS
AI is irrevocably altering customer service. This powerful technology is becoming integral to customer service frameworks, especially in contact center operations. It is transforming key aspects such as employee recruitment, training, empowerment and team performance evaluation.
AI is enhancing customer service by broadening and refining the use of bots for quicker resolutions, dealing with issues once managed solely by humans. While embracing the advancements brought by AI, it’s important to address concerns about its training, evaluation and impact on human staff.
How companies and the industry balance these concerns will be key to effectively using AI alongside human employees to improve customer service and support.
AI-Powered Recruitment: Potential and Concerns
AI-powered tools streamline recruitment, identifying candidates with the targeted blend of skills and cultural fit. These tools analyze resumes and interview responses with remarkable accuracy, significantly reducing errors and decreasing costs.
However, concerns arise regarding the potential for AI to perpetuate or exacerbate existing societal biases within the hiring process. Additionally, there’s a risk of over-reliance on automation, neglecting the value of human judgment and intuition in assessing potential employees.
Training with AI: Optimization and Questions
AI-powered training platforms are accelerating the speed at which new agents reach proficiency. These platforms evaluate individual strengths and weaknesses, customizing training for a more rapid transition into their roles.
However, questions remain regarding the potential for AI-powered training to create information silos, hindering knowledge sharing and collaboration among employees, including agents.
Furthermore, there are ethical considerations about employing AI to observe and assess performance during training, especially when these evaluations contribute to decisions about continued employment.
The Agent’s AI Partner: Collaboration and Concerns
AI is no longer a mere tool; it has become a valuable partner, offering real-time insights, knowledge and support to agents during interactions. This collaboration improves efficiency and accuracy, creating a more positive and productive experience for agents and customers.
Yet, there are concerns about generative AI potentially overstepping its role, producing responses or taking actions that may not adhere to company policies, compliance requirements, or ethical guidelines. Moreover, there’s a risk that heavy reliance on AI could diminish human agents’ critical thinking skills and problem-solving abilities.
Workforce Management: Accuracy and Data Quality
AI analyzes historical data and forecasts call volume with remarkable accuracy, empowering managers to optimize staffing schedules. This forecasting ensures efficient resource allocation and minimizes customer wait times. However, the effectiveness of AI’s predictions depends heavily on the quality and quantity of its training data.
Combining AI’s data processing with human insights about attributes that may deviate from past trends creates a balanced and accurate approach to forecasting staffing needs. This method enhances the precision of predictions by integrating large historical data sets with expected changes.
Call Quality Assessment: Insights and Ethics
AI listens to calls, scores agent performance objectively (based on training) and generates detailed feedback reports. This data-driven approach identifies improvement areas and fosters a continuous learning culture.
However, ethical concerns emerge regarding the potential misuse of the data. Additionally, there’s a risk of AI misinterpreting nuances in communication, leading to inaccurate assessments of agent performance.
Regular calibration of AI assessment tools to a set standard is necessary for maintaining their effectiveness. Targeted checks ensure these tools consistently adhere to this standard, thus ensuring their manageability.
Shared Responsibility: Human and AI Collaboration in the Call Center
AI’s arrival in the call center has undoubtedly improved efficiency and customer satisfaction. However, its impact goes beyond simply amplifying human capabilities. AI is now assuming important tasks previously handled by humans.
This shift in responsibilities presents both opportunities and challenges for the future of the contact center workforce. Contact center leaders must comprehend and navigate these evolving dynamics effectively.
Job Shift and Transformation: Upskilling and Reskilling for the Future
AI’s impact goes beyond simply amplifying human capabilities. AI is now assuming important tasks previously handled by humans. AI’s ability to automate repetitive tasks like data entry, scheduling appointments and handling simple inquiries is undeniable.
This automation will undoubtedly lead to some job displacement in the contact center industry. However, this doesn’t necessarily mean a bleak outlook for human employees. Instead, it signals a shift in the types of roles available.
As AI handles the routine, human agents will be free to focus on more complex tasks that require empathy, creativity and critical thinking. These roles could involve building deeper customer relationships, resolving intricate issues, and providing more personalized support. The need for flexibility and basic IT skills will likely increase across the human agent population.
This shift in responsibilities presents both opportunities and challenges for the future of the contact center workforce.
A Collaborative Future: Building Bridges Between Humans and AI
Despite AI’s increasing capabilities, human agents remain irreplaceable in delivering exceptional customer experiences. The future of the call center lies not in a competition between humans and AI but in a collaborative partnership.
By leveraging each of the unique strengths of both, contact centers can create an environment where efficiency and empathy work hand-in-hand. This strategy ultimately leads to a more satisfying customer and employee experience.
Transparency and Ethics: Ensuring Responsible AI Deployment
With AI’s increasing presence, contact center and CX leaders must commit to its responsible and ethical deployment. The ethical deployment includes being transparent about AI’s role in the decision-making processes. There should be safeguards to counteract potential biases in data and algorithms, particularly those impacting employment decisions. Additionally, it is important to ensure that AI tools augment, not replace, human judgment.
EVALUATING CHANGES TO THE COPC CX STANDARD
Before we evaluate changes, it’s important to establish a common understanding of the following aspects of CX operations and the COPC CX Standard:
- Artificial Intelligence (AI) – the technology enabling computers or automated robots (bots) to perform tasks typically done by humans. This technology is rapidly advancing and is a critical development in CX operations. This development is transforming the nature of work and agent responsibilities.
- Machine Learning (ML) – is a subset of AI that equips systems with the capability to learn and improve their performance autonomously, without direct programming. Instead, these systems evolve through analyzing historical data and past decisions. Machine learning is similar to an advanced form of human self-training, where continuous learning and improvement occur without explicit instruction.
- AI and Current Exhibit 1 Metrics – AI deployed into the performance of Key Customer Related processes (KCRPs) is measured in service, efficiency, sales and quality.
Critical to quality are measures of customer, compliance and business-critical errors, as well as contact resolution. Currently, several metrics measure the outputs of AI-supported conversational and non-conversational digital transactions. There are 13 metrics for digitally assisted transactions in Exhibit 1 of the COPC CX Standard.
- AI and Current Exhibit 2 Metrics – The Standard does not have pro-active control requirements/items for AI training as is found with the measurement of Key Support Processes (KSPs) for humans (Training Quality and Training On-Time), nor are there measures of effectiveness for tools supporting staff in the delivery of KSPs or KCRPs.
- AI and Training Accuracy – The Standard does not currently require measurement of AI Training Accuracy. Some recent practices have defined calculations assessing precision and recall as F Scores. This paper will not detail the use of the F Scores, leaving the potential use of AI accuracy measures to the COPC Standards Committee for review.
With the above in mind, the Committee is reviewing and evaluating AI’s impact on potential changes to the Standard.
- Terminology
- The vocabulary used in the Standard will be reviewed and updated to reflect AI’s changing role in CX operations.
- The Committee is assessing AI in customer-facing roles and how AI may perform all or part of Key Customer Related (KCR) jobs executing KCRPs. Issues include:
- Are there any new KCR jobs that need to be identified due to AI’s use in CX operations?
- Are there any existing KCR jobs that need to be updated or refined due to the use of AI in CX operations?
- These definitions and use of these terms, currently related to human roles, will be evaluated for how they account for instances where AI performs all or part of the function:
- Staff
- Customer Service/ Support Staff (CSS)
- Assemblers
- Shippers
- Collectors
- Processors
- Agents
- Trainers
- Planners
- Are there any new KCR jobs that need to be identified due to AI’s use in CX operations?
- The definition of Key Suppliers will be evaluated for inclusion of those organizations that perform or whose technology performs KCRPs or KSPs.
- The KSPs found in Exhibit 2 will be reviewed for any needed updates to clarify the role of AI in the performance of KCRPs.
- An example of a terminology change:
- In the context of contact centers where AI now performs tasks traditionally handled by human agents, redefining “key customer-related jobs” can be approached by acknowledging the evolving role of technology in customer service.
The COPC Standards Committee might modify the definition to encompass both human and AI roles, ensuring that it reflects the broader spectrum of tasks involved in providing customer service and support.
For example: “KCR jobs are those positions that either perform or directly manage staff performing KCRPs, which include tasks traditionally carried out by human agents and those now executed by AI technologies. These processes aim to provide effective and efficient customer service and support, utilizing a combination of human expertise and artificial intelligence capabilities.”
This definition recognizes the collaborative nature of customer service in contemporary contact centers, where AI and human agents often work together to deliver optimal customer experiences. It emphasizes integrating technology into customer-related roles, reflecting the industry’s changing landscape.
For customer contact operations, KCR jobs historically included human staff, including CSS or CSSs on the phone, using email or as web agents, collectors, telemarketers, mail/fax processors, and now may include AI-driven systems.
This definition encompasses the human staff who historically performed many KCRPs and expands to include AI-driven processes, acknowledging that AI can also contribute to or perform these KCR jobs.
- In the context of contact centers where AI now performs tasks traditionally handled by human agents, redefining “key customer-related jobs” can be approached by acknowledging the evolving role of technology in customer service.
- The vocabulary used in the Standard will be reviewed and updated to reflect AI’s changing role in CX operations.
- Metrics – The COPC Standards Committee identified the need to evaluate the Standard’s current Exhibit metrics to determine if any new metrics or modifications are needed. A subcommittee has been formed to assess how AI’s role may create the need for new metrics or changes to existing metrics required to ensure high-quality and reliable delivery of CX operational services.
- This will include a full review of applicable metrics in Exhibit 1 for KCRPs and Exhibit 2 for KSPs.
- A review of new ways AI impacts CX operations and the potential for new metrics noted a lack of measures for AI training and calibration.
- An example of a metric to consider:
- The F Score (or F1 Score) is increasingly used in the industry and may be a candidate for inclusion in the Standards. It is a metric combining Precision and Recall, which is particularly valuable in assessing the performance of AI systems in contact centers.
Given the dynamic nature of customer interactions, where accuracy and completeness are equally critical, the F Score offers a balanced evaluation by considering both false positives and false negatives.
In the context of contact centers, an optimal AI solution should not only swiftly identify relevant information but also minimize errors, ensuring accurate responses to diverse queries.
The F Score’s ability to strike a balance between precision and recall makes it a suitable metric, enabling organizations to gauge the efficiency and effectiveness of their AI systems in handling customer inquiries, ultimately contributing to enhanced customer satisfaction and operational efficiency.
- The F Score (or F1 Score) is increasingly used in the industry and may be a candidate for inclusion in the Standards. It is a metric combining Precision and Recall, which is particularly valuable in assessing the performance of AI systems in contact centers.
- This will include a full review of applicable metrics in Exhibit 1 for KCRPs and Exhibit 2 for KSPs.
- Use Case Analysis – Monitoring the use of AI, with an added focus on generative AI, in the performance of KCRPs.
- Identifying use cases that do/may impact end-user customer satisfaction.
- Understand the role of AI and those that manage the use and activity of AI in digitally-assisted transactions and support of human-assisted transactions.
- Are these “transaction” definitions/terms accurate? Refer back to the terminology subgroup.
- Are the roles that train and manage AI identified and defined for consideration as KCR jobs?
- Is there a need for calibration among those that assess and tune the performance of AI? What happens when AI is supposed to assess itself?
- Are these “transaction” definitions/terms accurate? Refer back to the terminology subgroup.
- Noting the expanding use of AI across all categories of the Standard impacts how many/most companies perform activities governed by the enumerated items of the Standard.
- Many examples of AI use cases are taking hold across the industry. The Committee will look at a full range of use cases, from automated support to agent assist, service journey management and workforce management, to list a few.
- Identifying use cases that do/may impact end-user customer satisfaction.
Managing Changes to the COPC CX Standard
Any time the COPC Standards Committee changes the Standard, the group is methodical in ensuring clear and consistent communication to the user community. The potential changes surrounding AI is no different and the Committee’s approach will include the following:
- Keep the output of processes and impact on end-user satisfaction as the focal point, avoiding the creation of prescriptive requirements on how AI functions/processes work. (While interesting, we care only that it can be managed to high quality, measurable and manageable delivery.)
- Changes to the Standard must be able to be implemented by a broad set of users worldwide.
- Where needed, the Committee can publish communications before new versions of the Standard. These communications enable visibility into the work and direction of the Committee related to the use of the Standard.
- Using and communicating best practices, found in the COPC Best Practices Handbook, is an important way to trial changes for adaptability and effectiveness. This approach also allows fast-changing technology environments to mature before creating requirements impacting all users of the Standard.
COPC Inc. and the COPC Standards Committee are committed to informing the user community about these and other potential modifications. Since its establishment in 1996, the Committee has consistently worked to ensure that the Standard keeps pace with the industry’s evolving dynamics. As the application of AI in enhancing customer experience rapidly advances, the Standard will adapt accordingly to these developments.