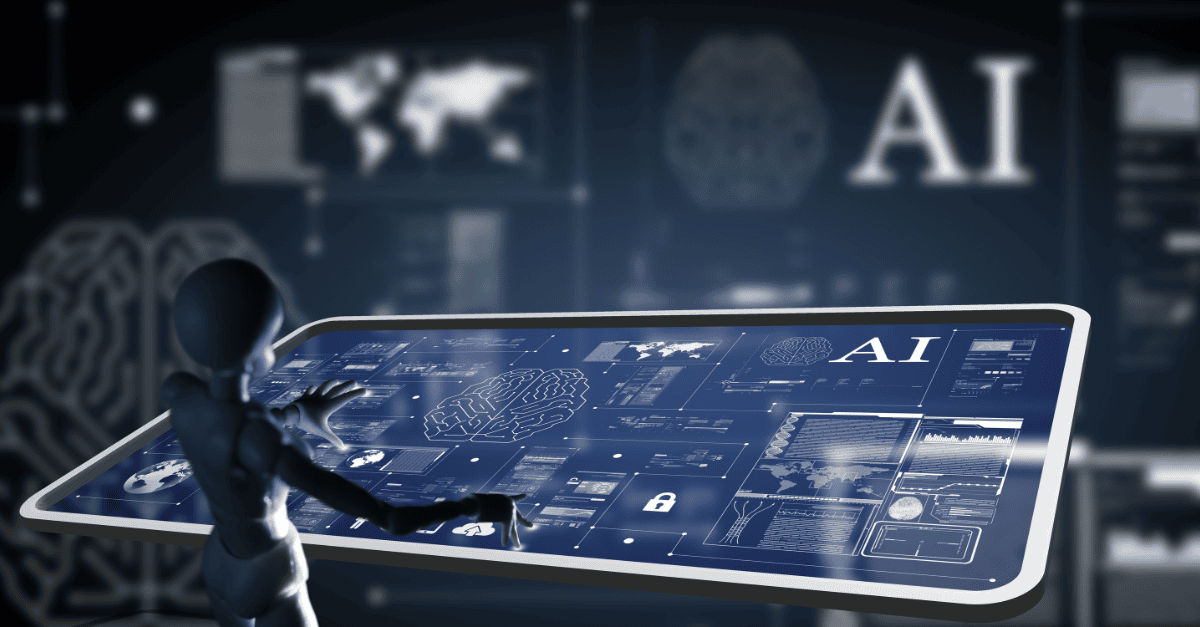
April 2, 2024
Artificial intelligence (AI) is rapidly transforming the customer experience (CX) industry, offering businesses new opportunities to enhance their operations.
This blog article discusses the importance of employing multiple types of AI, implementing effective controls and guardrails, and adapting specific roles within the CX organization. With these strategies, businesses can maximize the benefits of AI and thrive.
AI is Ideal for the CX Industry
The CX industry is embracing custom AI solutions for compelling reasons, and it’s no surprise why. To start, this industry often involves repetitive tasks that can be automated, making it an ideal environment for AI implementation. Additionally, the high volume of interactions handled by CX professionals creates fertile ground for AI-driven efficiencies.
Moreover, the industry’s familiarity with leveraging IT systems makes it open to integrating AI technologies seamlessly. Lastly, the wealth of data generated in the CX industry provides invaluable insights for businesses looking to gain a deeper understanding of their customers.
The Many Facets of AI in CX
Applying AI in the CX industry unlocks many opportunities to enhance daily operations and customer interactions. However, it’s important to recognize that no one-size-fits-all AI solution exists. Different AI methodologies, technologies, and approaches are available for specific use cases.
Historically, the CX industry has focused on optimizing agent performance and support functions, leading to the integration of AI in these areas. This integration empowers agents with timely and accurate information, ultimately improving the overall customer experience. More recent evolutions within AI make it feasible for AI to interact directly with customers through all digital platforms—not just chat, as seen so far.
Moving Beyond Support Functions
AI’s role in CX is evolving beyond support functions. The future lies in AI delivering the entire customer journey and elevating customer service experiences. As a result, AI solutions are diversifying and becoming more specialized, catering to different aspects of the CX landscape. The AI capabilities are moving into carrying out the actual work of agents as well, including communicating with customers.
Understanding Generative and Analytical AI in CX
To fully understand the spectrum of AI in the CX industry, it is helpful to categorize AI into two primary types: generative AI and analytical AI. Both types have unique applications and play a significant role in shaping customer experiences and uncovering valuable insights.
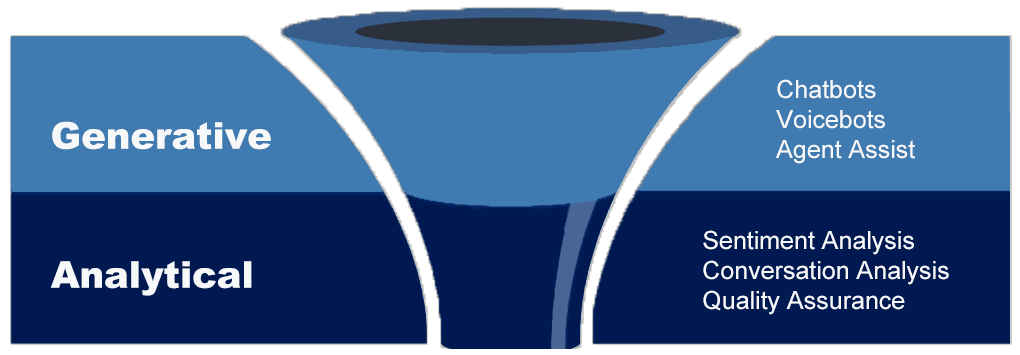
Generative AI: Personalized and Engaging Experiences
Generative AI focuses on delivering customer journeys and creating personalized interactions. It utilizes algorithms to generate seemingly new content, enabling businesses to engage customers through chatbots, virtual assistants, and AI-driven customer service platforms.
It’s important to note that generative AI operates based on probabilities, simulating human responses by analyzing historical patterns and related data.
Analytical AI: Uncovering Insights from Customer Journeys
Analytical AI focuses on measuring and comprehending customer journeys. Analyzing vast amounts of data identifies patterns and provides valuable insights for informed decision-making. Conversation analytics, speech analytics, sentiment analysis and automated quality assurance are tools used in analytical AI. These tools leverage data and algorithms to uncover previously inaccessible insights due to manual analysis limitations.
For example, AI enables organizations to move beyond traditional sampling and analyzes complete datasets. This capability opens possibilities for in-depth analysis in areas like conversation analytics, speech analytics and sentiment analysis.
Harnessing Custom Data for Control Over Personalized Customer Experiences
Generating accurate responses to customer inquiries requires relevant and specific data. Day-to-day operations in the contact center generate much of the data used in the CX industry. Structured processes for gathering and treating this data are necessary. Just as with custom data used for training AI models, the data gathered for operational purposes requires understanding, representation and completeness.
Generative and analytical AI offers unique advantages in the CX industry. By harnessing custom data, businesses can enhance customer experiences, gain valuable insights, and make informed decisions to stay competitive in the evolving customer experience landscape.
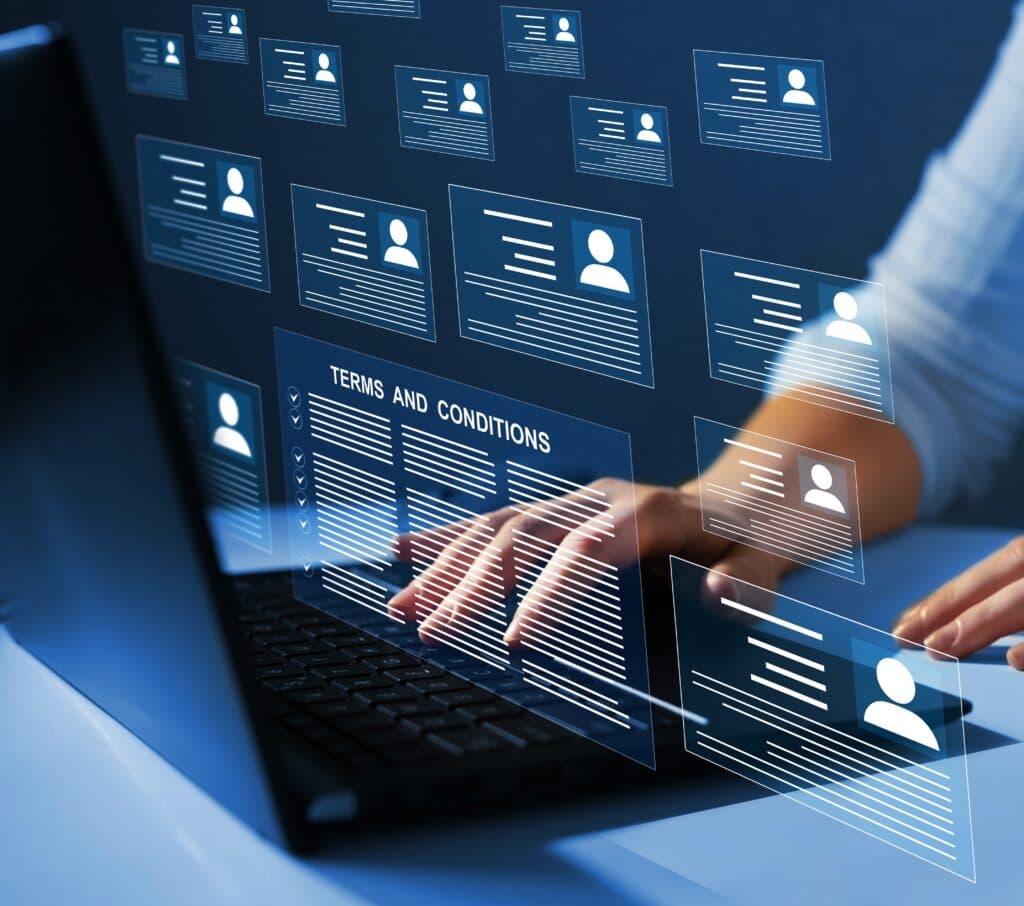
The Evolution of Generative AI
While generative AI, like ChatGPT, can provide answers based on publicly available information, controlling outcomes is essential. Restricting the scope of generative AI responses enhances brand consistency and establishes a unique connection with customers.
Training Analytical AI
Analytical AI focuses on understanding customer sentiment and uncovering insights about specific actions or behaviors that elicit positive or negative responses. Businesses gain valuable insights into customer reactions and preferences by training analytical AI on a wide range of symptoms or behaviors and breaking down the analysis into targeted areas.
Applying the underlying technology and knowledge database used for sentiment analysis in a controlled environment enables a deeper understanding of specific customer preferences. Businesses can implement generative AI in a controlled environment by utilizing custom data. Training the AI model with specific datasets relevant to the company ensures that the generated responses remain relevant, accurate and aligned with the brand identity.
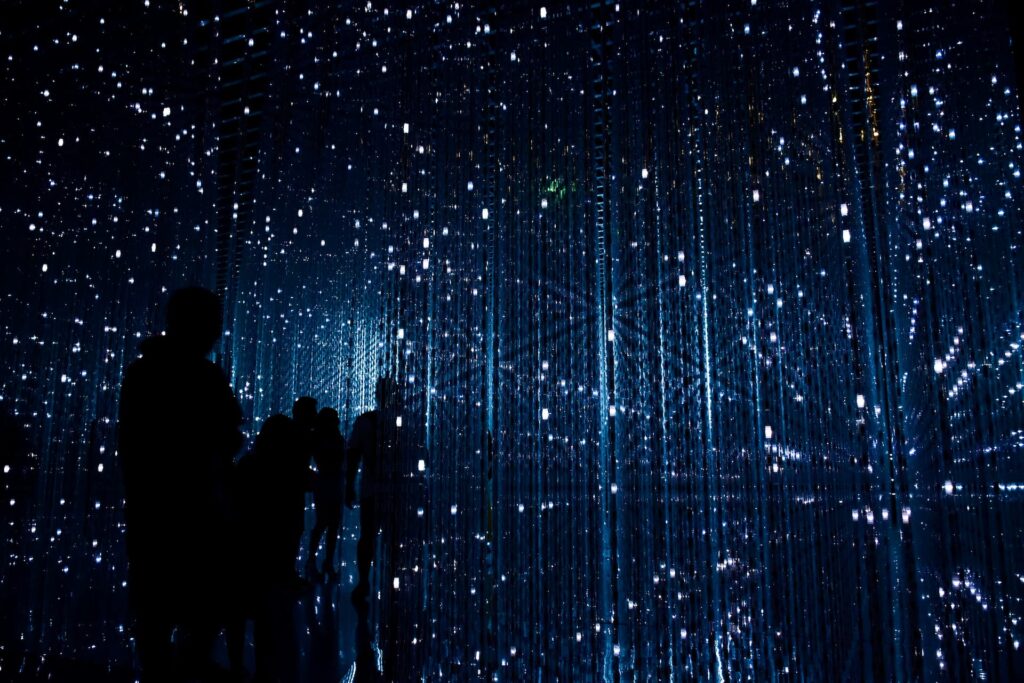
Related
CX Technology Consulting
Fusing technology and expertise to design and deliver exceptional service journeys.
Generating Custom Data for Accurate Responses
By adopting a systematic approach to data handling, businesses can unlock AI’s true potential and generate valuable insights to enhance customer interactions. Customizing data plays a vital role in developing responses with generative AI. It involves emulating the training process of a highly skilled agent. Similar to training a human support agent to handle customer inquiries, AI models require training in using relevant datasets. There are two key components to consider when customizing data for generative AI.
To start, you need to teach the AI how you want the answers delivered, specifying the desired tone or approach, just as you would train a human agent. Then, carefully curate the data to ensure accurate outcomes.
In the case of analytical AI, predictability is essential. The AI needs to analyze and process data, identify patterns, and understand the context within those patterns. It must distinguish between important and unimportant words in a sentence to generate accurate and relevant responses. Building a custom dataset may seem daunting, but the good news is that you likely already have the data you need.
Leveraging existing CX data allows you to train the AI model using real-world examples and customer interactions specific to your organization. Preexisting data ensures that the AI understands the unique nuances of your business and can provide accurate and context-aware responses. By harnessing custom data, organizations can optimize the performance of their AI systems and deliver enhanced customer experiences.
Customizing AI for Enhanced Outcomes: Training and Managing Data
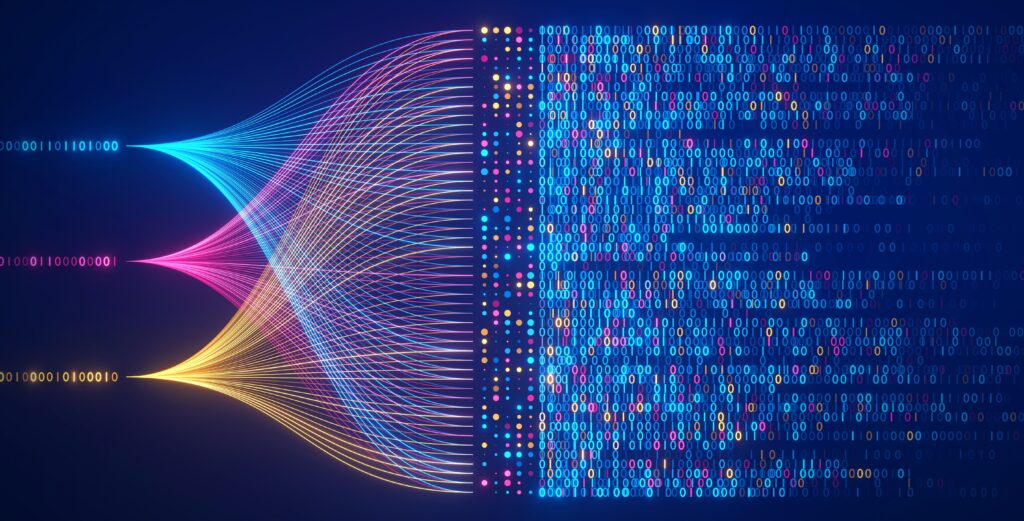
Custom AI doesn’t involve starting from scratch; it focuses on modifying existing datasets to meet specific needs. By leveraging generative data, algorithms and knowledge, we can tailor AI systems to deliver desired outcomes unique to your business. This approach offers several advantages, enabling the application of advanced technology to achieve accuracy and efficiency.
The Importance of Accuracy in Predictions
When it comes to predictions that require high-level accuracy and mathematical calculations, custom AI plays a significant role. Obtaining the correct answer or support guide is crucial for maintaining predictability. Ensuring accuracy means having individuals who understand what constitutes the right answer and can effectively organize the data.
For the best results, it is imperative that the people who organize the data and train AI models understand not only data – they need to understand CX as well.
Within the CX industry, there are already existing roles where data knowledge needs to be coupled with operational knowledge. These individuals are forecasters. They closely monitor various factors, predict volumes and average handling times (AHTs), develop capacity plans, etc.
It is a similar environment you want to create when applying it to AI within the operations. Put simply, you will avoid the risk that AI has a large degree of variation in its predictive models, enabling the AI to provide the correct response far more often than otherwise.
Skewing Data Towards Known Correct Answers
In the context of knowledge, it’s not about dumping all available information into a database and assuming it’s sufficient. Instead, the focus is on skewing the data towards known correct answers, increasing the probability of finding accurate responses.
We can enhance its effectiveness by guiding AI to focus on certain directions and avoid others. However, you need individuals close to the organization who understand the data, generate it, and determine what needs to be left out. What we put into the AI model ultimately determines its performance.
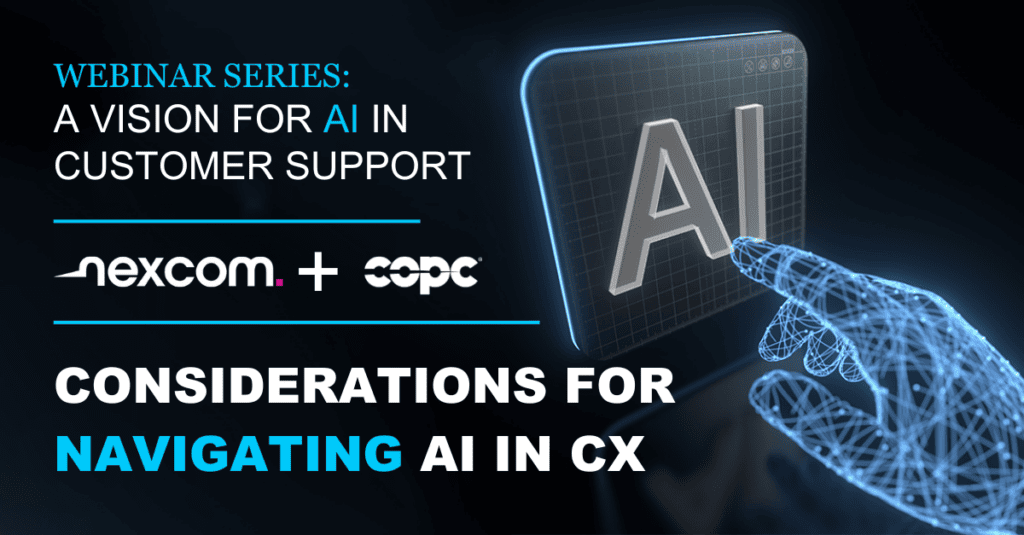
Webinar:
Considerations for Navigating AI in CX
This session explores strategies for seamlessly incorporating AI into customer service and shaping the future of customer experiences using an AI framework. It also highlights the pivotal role of high-quality data in enriching customer experiences.
Building Effective Data-Utilization Processes
Data management is critical in AI, and building effective data-utilization processes is essential. Linking knowledge database articles with correct answers or preferred methods is helpful. Organizations can measure the frequency of article usage in conjunction with speech analytics to determine their relevance.
Quality monitoring data is also instrumental in distinguishing between right and wrong approaches. While manipulating data for accuracy may not seem popular, it is necessary to recognize that using raw data without manipulation would yield results similar to those achieved by contact centers today.
Various roles within the CX industry, such as quality assurance (QA) professionals and trainers, can contribute to this process. QA professionals deeply understand quality data and can identify bugs or inconsistencies. At the same time, trainers can provide valuable insights based on their expertise in using tools and training people.
Organizations can identify the right and wrong aspects by compiling data from these sources and involving knowledgeable individuals. This approach aligns with the evolving roles within the CX industry, where utilizing expertise is encouraged for effective data management.
Maintaining Skepticism and Accuracy in Data Usage
To avoid such pitfalls, organizations must evaluate data sources critically and consider multiple perspectives. Tagging the knowledge database, conducting polls, leveraging quality monitoring, or analyzing exam results can contribute to the normalization process and help distinguish between correct and incorrect answers. By ensuring data accuracy, organizations can build reliable AI models that deliver accurate outcomes.
Mindlessly trusting data without questioning its accuracy can lead to erroneous assumptions. Relying on inaccurate data sources to train AI models would yield flawed outcomes.
Empowering Traditional CX Roles in the Age of AI: Nurturing Human Expertise
In the CX landscape, AI is often seen as a potential replacement for human agents. However, the paradox lies in that agents are inseparable from practical training and AI system guidance. Their specialized knowledge is necessary for designing AI solutions that deliver desired outcomes, and their involvement in fine-tuning AI models leads to continuous improvements. Therefore, organizations can support the digital side of CX operations by elevating traditional roles.
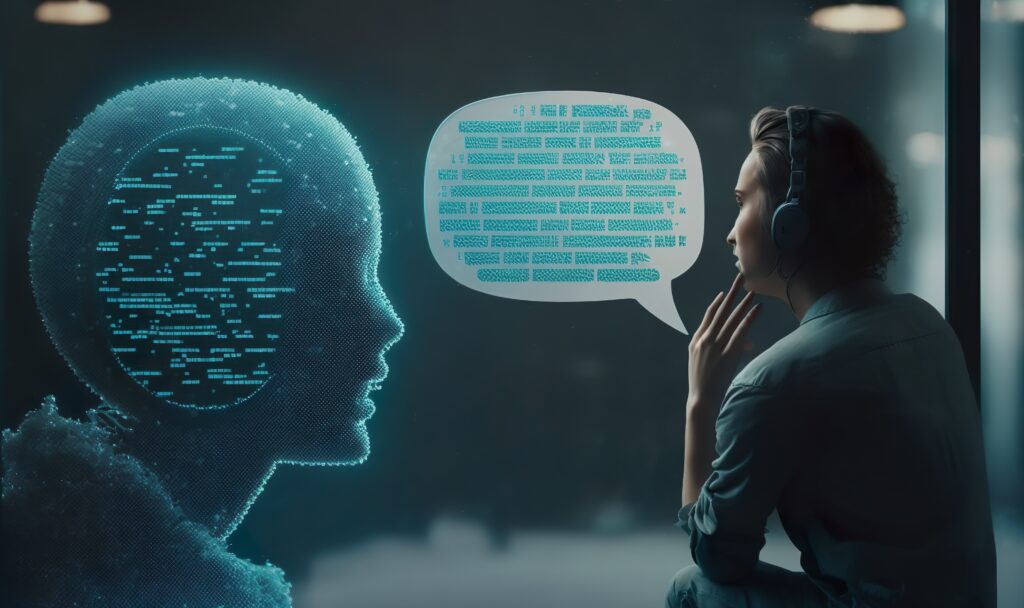
The Importance of Quality Work and Agent Expertise
As the demand for AI assistance increases, it is crucial to reinforce the focus on training and quality monitoring. However, it is also necessary to empower these roles to expand their knowledge and apply it to tasks like data sanitization.
Despite AI’s growing presence, the importance of quality work and agents’ pivotal role in delivering exceptional customer care remain unchanged.
Fostering Collaboration and Inclusivity
Emphasize the continued relevance of traditional roles and involve them in shaping the organization’s future to foster a culture of collaboration and inclusivity. As with other introductions of technology, organizations should plan and act accordingly. Organizations are not replacing agents. Still, the job will change over time as AI and other technologies evolve, and there remains a need for staff to be present for AI to function properly. This shift creates a sense of purpose and reassurance about their place within the organization.
Expanding Agent Contributions to AI Training
Beyond their primary responsibilities, agents will contribute to AI training, marking a significant change in the CX industry, where maintaining quality in work is crucial to the correctness of AI models. Ensuring that AI consistently delivers the correct answers requires leveraging the expertise of well-trained agents.
Effective Data Management and CX Understanding
Effective data management is the foundation of good AI. Data analysts must deeply understand the data sources and the purpose behind collecting that data. Those working in analytics teams should undergo a rigorous sanitization process and understand the CX landscape. This holistic perspective ensures that compiled data aligns with CX goals and objectives.
Enhancing Customer Experience through AI and CX Integration
Centralized teams can provide valuable insights, but understanding what constitutes not just a correct answer but a good one, can be lost without involvement from CX experts. IT professionals should be exposed to frontline customer care to understand the CX landscape better.
Collaboration and understanding across teams are crucial to bridging the gap between industry professionals and the mathematical expertise required for effective AI implementation.
Real-Life Examples of AI Customization for CX
Real-life examples highlight the significance of CX understanding in AI implementation. Collaborating with the CX team allows AI systems to provide comprehensive responses that address customer needs, engage conversationally, and offer further assistance. Customizing AI models through continuous training and a deep understanding of customer needs leads to exponentially improved AI systems.
Maximizing AI Effectiveness: Monitoring, Training and Embracing CX
Monitoring and reviewing AI systems for accuracy ensures their reliability. Establishing a monitoring process that includes common and obscure questions allows us to assess the AI’s ability to provide accurate answers. Continuous training and validation of correct and incorrect responses enable AI systems to recognize and differentiate between them.
Evolving Roles and Active Participation
Organizations must understand the importance of ensuring that agents possess the necessary skills and knowledge to deliver exceptional customer experiences. Agents’ active participation in bug finding, reviewing the knowledge database, and intentionally triggering incorrect answers during AI testing generates valuable data for data analysts. This dynamic shift transforms the agent pool from an industrialized approach to a collaborative process that values quality and actively contributes to AI improvement.
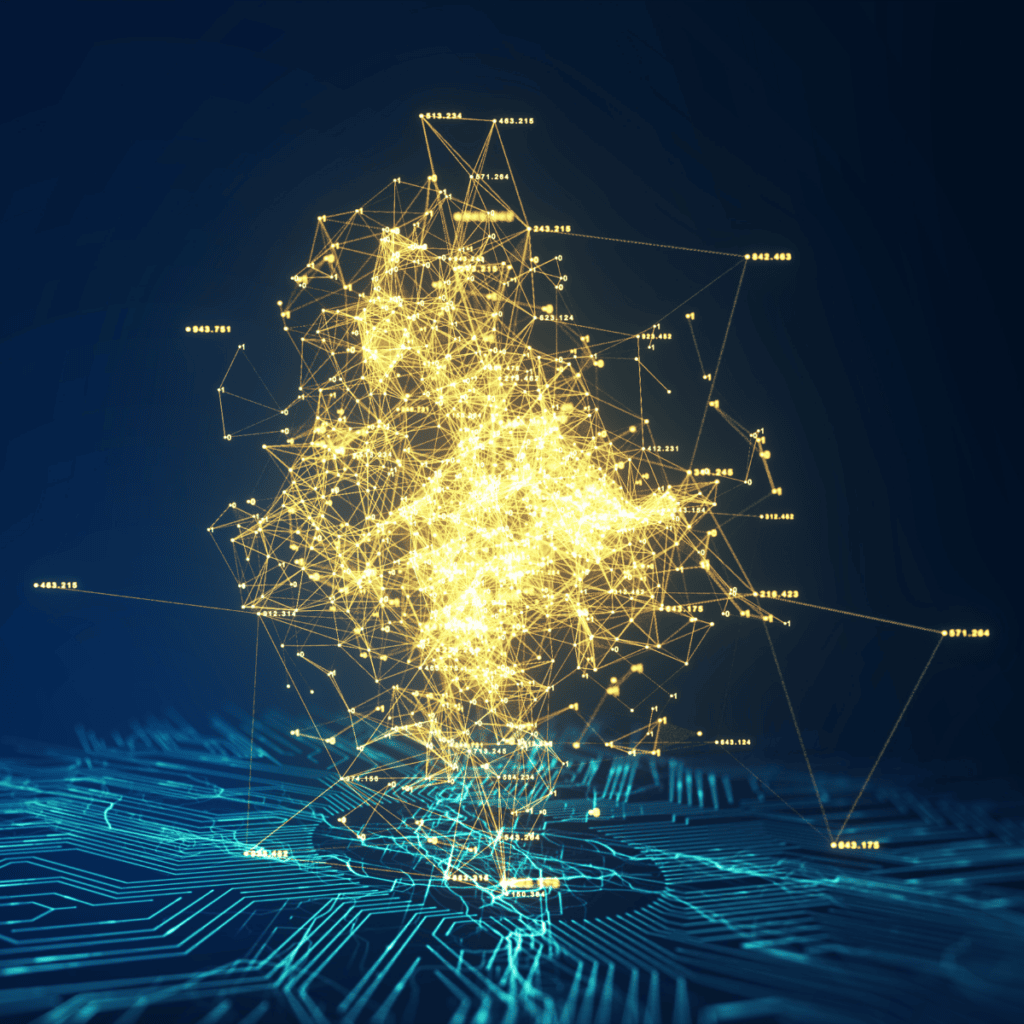
Conclusion
AI has proven to be a game-changer in the CX industry. By leveraging the power of AI, businesses can enhance their operations and deliver exceptional customer interactions. However, there are several considerations that organizations should keep in mind when implementing AI in CX.
Firstly, different types of AI solutions cater to specific use cases. Generative AI focuses on delivering personalized and engaging experiences, while analytical AI uncovers insights from customer journeys. By understanding the unique applications of each type, businesses can leverage AI effectively.
Secondly, harnessing custom data is crucial for accurate and context-aware AI responses. By training AI models with relevant and specific datasets, organizations can ensure that the generated responses align with their brand identity and customer preferences. Effective data management processes and involving knowledgeable individuals, such as quality assurance professionals and trainers, contribute to the accuracy and reliability of AI models.
While AI plays a significant role in transforming CX, it is important to remember that human expertise remains essential. Exceptional agents are crucial for training and guiding AI systems, and their involvement leads to continuous improvements. Traditional CX roles are malleable and can support the digital side of CX operations and empower agents to contribute to AI training.
In summary, AI presents exciting possibilities for the CX industry. By embracing multiple types of AI, implementing effective data management practices, and nurturing human expertise, businesses can unlock AI’s true potential and deliver exceptional customer experiences.
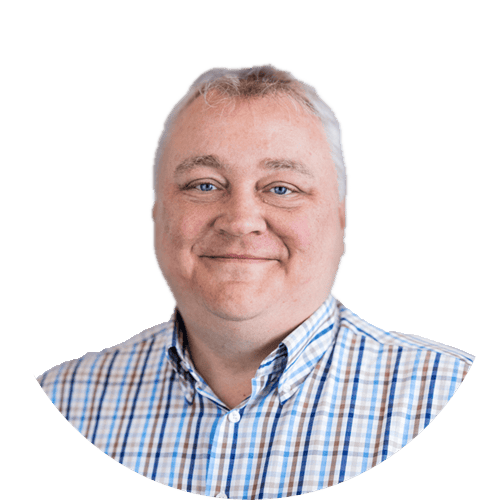
Guest post by Rolf Adamson, CEO at Nexcom A/S